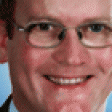
It is generally understood that quality information is an enabler for cost cutting, risk reduction and revenue enhancement. However, many companies have different approaches to managing their corporate information asset. These approaches range from ad hoc and tactical projects to meet specific goals, to strategic imperatives that seek to embed data quality principles across the corporate architecture.
This makes it difficult to know where to start, what is effective, or whether the company is on track for success in meeting the data management challenge, and which of these myriad approaches is best.
For many companies, data management remains a reactive process, with individual project teams or business areas identifying specific data issues that are impeding the ability of the project to achieve a specific goal. Short-term measures may include tactical data cleansing initiatives, with or without a tool, and typically do not take corporate standards and goals into account.
Data dilemmas
For example, the marketing department may embark on a short project to clean a customer marketing list to target a new segment. This project could deliver real benefits, increasing the success of the resulting campaign. However, in most projects of this nature, the root causes of data quality problems are not addressed, such as customer details that are not updated on a regular basis. The result - in a few months' time the data has deteriorated again and another project must be initiated.
Another common example is that data is transformed as it is loaded into the data warehouse in an attempt to improve the consistency of information used for core reporting. This contributes to the reality that operational reports, derived directly from the source systems, can give different answers to those reports derived from the data warehouse. Which report is correct? If business cannot trust a report, it will typically stick with the report it knows, even if it is wrong.
This approach results in rework and unnecessary expense. The problems are never really solved, so data cleansing efforts tend to focus their limited resources on a few applications, in a never-ending cycle of rinse and repeat.
Companies that recognise this waste are shifting to a new paradigm based on the principle that sustainable data quality improvement requires a more mature approach. This entails a metamorphosis from existing chaotic approaches to a more proactive approach that incorporates data quality metrics, standards, shared master data and data stewardship.
An enterprise-driven focus on data quality management requires data management principles to be embedded in the corporate culture, and this needs to be driven both top-down and bottom-up.
Bottomed out
Any enterprise change initiative will, ultimately, require executive support if it is to succeed, but this does not in itself guarantee success. The e-toll project in Gauteng is an excellent example of a project that has solid top-down support, but is suffering from a severe lack of buy-in at the bottom.
A data quality metamorphosis cannot be achieved in one step, just as a caterpillar does not become a butterfly without a number of changes.
A data quality metamorphosis cannot be achieved in one step, just as a caterpillar does not become a butterfly without a number of changes. A purely top-down approach is based on the belief that top management must provide funding and political capital in order to drive change. Without evidence that a new approach will deliver business value, management is unlikely to provide this support.
Similarly, small changes at an operational level will have less resistance from the bottom, but can have long-term benefits. A simple example is to explore how data needed for one project may also benefit or impact other users. This understanding of the enterprise impact of poor quality information can lead to a pooling of existing funds in order to achieve a broader, more sustainable goal.
Of course, any change cannot be regarded as successful without measurement. Changes must be measured in terms of both their business impact and their sustainability, ie, how much revenue was derived from cleaning this data, and how much was saved by reducing the need to intervene again.
It is important to build on early successes in a sustainable way to reach data management maturity. The initial understanding of where a company is will allow it to identify opportunities for incremental improvement, while providing the business case and political capital to justify further investment. Data quality is not a project and should form part of a sustainable data governance programme.
Click here to take this free survey - IT Management Use of Data Quality - to assess the effectiveness of your approach against those taken by over a thousand IT and business professionals across the globe.
Share