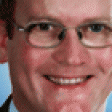
'Big data' is a buzz term, spawning a host of new technologies and comment. One of the challenges for implementers is that there are conflicting views as to what big data is. Everyone agrees, though, that big data is about volume.
The principal focus of most commentators, and most technology solutions, is related to dealing with this volume. Traditional relational databases were primarily designed to enable easy searching and reporting on data, but anyone who has run a query against a large dataset knows it can take hours, or even days, to obtain results. Newer technologies, such as the open source Apache Hadoop or Software AG's Teracotta platform, are designed to support large volumes via a distributed architecture and in-memory data management.
The real challenge of big data, however, is not volume, but structure or the lack thereof. Big data comes in a variety of formats, from machine-generated feeds, to telecommunications call records, to various free format Web sources and business communications.
An example of where big data is expected to add significant value to business analytics is social intelligence, providing the ability to mine social media to analyse clients' or prospects' feelings about a company, products and brand. Why extrapolate opinions from focus groups and surveys, the thinking goes, when clients' opinions are captured on Facebook, Twitter, HelloPeter and similar sources? Analysis of the entire client group removes the need for assumptions - leading to more accurate planning and the ability to respond rapidly to emerging trends.
Six feet under
The real challenge is that valuable, relevant information is buried amid a massive volume of clutter. Relevant content must be pulled from unstructured text fields such as blog posts, e-mails, letters and the like, and linked together across multiple user profiles and applications.
Common sense suggests that filters must be applied to reduce volumes - there is no value investing in infrastructure to store irrelevant data. These filters can be incorporated through various data quality tools and other business rules to deliver the ability to search for relevant information.
The requirement then is to ensure big data is fit for purpose - a data quality problem. Of course, free format text data is not restricted to the Internet. Increasing volumes of data are being created by technology that generates and stores information.
In the age of big data, quantity of data is far outstripping quality of data.
Take, for example, smart meters that track real-time utility consumption. Another example is global positioning and telemetry systems in vehicles. They all generate volumes of data, which mean nothing if they are not linked to a source such as a customer. By creating this link or association, utility companies can create a profile of the customer based on their consumption of electricity.
Transforming meaningless big data into intelligent and contextualised information allows organisations to better plan demand, manage risk and improve customer services, further enabling the organisation to reach its business goals.
Too much
Even business correspondence, such as e-mails, letters and facsimiles, can hold valuable and time-critical information or instructions, which can easily be overlooked due to sheer volume. These oversights lead to additional administrative costs, or may even result in legal liability, if charges are not responded to timeously.
In the age of big data, quantity of data is far outstripping quality of data. However, underpinning the success of creating actionable intelligence out of big data is data quality. Data quality solutions bridge the gap between traditional business analytics and big data analytics - delivering value today on existing data sets. The same technologies can be deployed tomorrow against social media sets, to ensure confidence that real insight will be gained from investments in big data infrastructure.
Data quality can also assist to control costs within an organisation. Essentially, storing data costs money due to the requirement for hardware. This is necessary in order to keep backups, archive information and retain the information for a number of years, usually driven by governance and compliance. Unnecessary costs will be incurred should a user store, for example, all Facebook comments, due to the inability to filter relevant information. Data quality tools should therefore be applied to filter and discard useless and irrelevant data from information that delivers business insight. As data volumes continue to grow exponentially, this function will become increasingly important and prevent the cost of infrastructure from spiralling out of control.
Whether big data is just about volume, or about volume and variety, data quality will remain critical to deriving value. When planning for big data, don't forget to plan for data quality.
Share