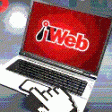
The ETSI Securing Artificial Intelligence Industry Specification Group (SAI ISG) has released its inaugural group report, ETSI GR SAI 004, which gives an overview of the problem statement regarding the securing of AI.
ETSI SAI is the first standardisation initiative dedicated to securing AI.
In the report, the problem of securing AI-based systems and solutions is unpacked, with a focus on machine learning (ML), and challenges in terms of confidentiality, integrity and availability at each stage of the ML lifecycle. In addition, it examines a few of the broader challenges faced by AI systems including bias, ethics and ability to be explained.
Several different attack vectors are outlined, as well as a number of incidents of real-world use and attacks.
Alex Leadbeater, chair of ETSI SAI ISG, says there are many discussions around AI ethics but none surrounding the standards need to secure AI.
“Yet, they are becoming critical to ensure security of AI-based automated networks. This first ETSI Report is meant to come up with a comprehensive definition of the challenges faced when securing AI. In parallel, we are working on a threat ontology, on how to secure an AI data supply chain, and how to test it,” he adds.
According to Leadbeater, in order to pinpoint the challenges involved in securing AI, AI had to be defined. The ETSI group defined AI as the ability of a system to handle representations, both explicit and implicit, and procedures to perform tasks that would be considered intelligent if performed by a human.
He stresses that while this definition covers a wide range of possibilities, a limited set of technologies are now becoming feasible, driven mostly by the evolution of ML and deep-learning techniques, as well as the wide availability of the data and processing power needed to train and implement such technologies.
A slew of approaches to ML are in common use, including supervised, unsupervised, semi-supervised and reinforcement learning, he says. “Within these paradigms, a variety of model structures might be used, with one of the most common approaches being the use of deep neural networks, where learning is carried out over a series of hierarchical layers that mimic the behaviour of the human brain.”
Moreover, various training techniques can be employed, such as adversarial learning, where the training set contains samples which reflect the desired outcomes, as well as adversarial samples, which are meant to challenge or disrupt the expected behaviour.
Although the term AI was coined in the 1950s, he says ithe report reveals how much it has evolved over the last 70 years, with cases including ad-blocker attacks, malware obfuscation, deep fakes, handwriting reproduction, human voice and fake conversation.
Share