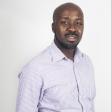
One may wonder about the data that is collected, processed and stored during normal business operations, which ends up in data centres and not used for any esteemed purpose. Such data may also reside in storage technologies considered obsolete or offline in nature. This is known as dark data.
A large number of write-ups on dark data focus on entirely unused data sets, such as server logs, mobile geolocation details or Web click-through details. Dark data is mostly associated with unstructured data where in excess of 90% of the data is not used for various reasons by companies. However, this does not lessen its importance in the context of business value.
It caught my attention that there are over 65% of attributes within used data sets that are not analysed, which could bring additional context or permutation to already used attributes to derive additional economic/operational opportunities.
To illustrate, I asked three new customers to point out three of their data marts they could not do without. I then looked at two of the common marts and reverse-engineered them back to the source. Note, these were built to satisfy the customers' requirements and still fulfil their needs to date.
About 65% of the attributes and measures in the source table did not make it into their data mart. Within that 65%, about 35% were not known to business users and were assumed to be system control logs of little value, while the rest of the 30% were known attributes, simply ignored because they are perceived to be of no value. The actual percentage of unused attributes goes even higher when unpacking the "overloaded" columns, such as XML and/or delimited content fields (could be as high as 90%). This is in line with the findings of research firm International Data Corporation, which reports up to 90% of big data is dark data.
Pleasantly surprised
On investigation and acquiring thorough understanding of what those additional 65% attributes were and how they related to the current system processes, business users quickly realised what a goldmine those were. Business cases started flowing and decades of unanswered questions became apparent. It was as if a box full of toys was opened to a 10-year-old child outside the Christmas giving period - imagine the excitement. They even started realising unused features they never knew existed within their operational system. These are features they could use to provide new and flexible products to increase revenue. All these were hidden in the above 65% attributes I call "intrinsic dark data".
Business cases started flowing and decades of unanswered questions became apparent.
Ben Austin, in his article: Why organisations should care about dark data, compared the scenario of uncovering new use cases to finding a R50 note in the pocket of a pair of pants that hasn't been worn in a while. Again, imagine this as a 10-year-old child.
Intrinsic dark data is a subset of used data attributed as unused, which usually constitutes the biggest portion of the total volume of used data. Intrinsic dark data is not usually analysed, understood or processed because of various reasons by organisations, and its business value is unqualified.
To this end, it's apparent that most data elements can be used to understand and/or improve something about the way a company does business. Some of these attributes are not necessarily genuinely intrinsic dark data, but rather, additional tags used in some transactional decision point by the system that generated the data - items that revenue assurance representatives need to be able to audit systems accurately. In a data warehousing environment and its nature of an open-ended audience, to ignore these tags is perfectly fine for one division, but might be unacceptable for the other departments. The purpose of domain-specific data marts was created for this very purpose - conflicting requirements.
Companies should always ask questions about the meaning of data attributes in system terminology, and possibly marry those to business processes. Data attributes becomes "intrinsically dark" only if not used, and can only be used once understood. Attributes that are acquitted of usefulness, although hard to believe, can be eliminated from the database to recover occupied space (and save on costs for space). Companies should make an effort to audit and prune dark data in general.
It is imperative to acknowledge that intrinsic dark data (unanalysed data attributes) may contain undiscovered, important insights and represents an opportunity lost, or even a pointer to the breakthrough companies have been searching for.
Share