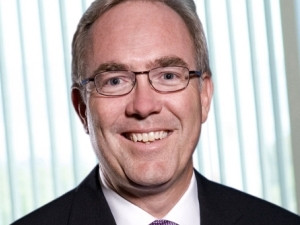
Artificial intelligence (AI) seems to have travelled from sci-fi fantasy to boardroom hyperbole at warp speed in no time at all.
While it's not surprising that a future working environment with fully autonomous thinking and acting robots is a topic that captures imaginations, trying to work out the relevance of AI to today's manufacturing environment is somewhat less clear.
Erik Johnson, chief architect, Epicor Software, helps to debunk some common myths around AI and put the hyperbole into some context for manufacturers trying to work out its relevance today.
Q: Can you help define AI for us?
AI is already an over-hyped technology within business. So, before I cover what it is, it might also be helpful to separate it from what it isn't. The following areas are often confused with AI. They have similar capabilities but aren't actually the same.
Predictive analytics means using historical data to predict what future data might look like. Sometimes this is easy, like finding that demand for a product is seasonal. But it's usually more complex. For example, demand in a particular region can also depend on weather. So, if you can combine historical sales and weather data, you can use long-range weather predictions to make a more accurate sales forecast. Predictive analytics is a very well understood branch of mathematics and has been used in supply chain scenarios for years.
Machine learning is the capability for an algorithm or program to improve over time. This can be achieved by filtering out data that leads to inaccurate conclusions, improving the algorithm itself, or by increasing the quantity of data used to determine an outcome. 'Unsupervised' machine learning is where the system tracks whether the outcomes were as expected and improves itself automatically. A good example of unsupervised machine learning is a search engine that also records which results a user accepts and modifies itself to put similar results at the top of the results for future searches. There is a lot of confusion about what constitutes machine learning verses artificial intelligence. AI is the broader term for something that constitutes 'smart' systems and machine learning is a subset of approaches, where machines use data to learn and reason and get better over time.
Artificial intelligence is a system that can reach the same (or better) conclusions as a human from the same kinds of inputs. Many early AI systems were built to analyse photographs to see if the computer could recognise people or objects, identify what the subjects are doing, and ultimately determine the consequences or next actions. This kind of work has led to the development of self-driving vehicles, which is an advanced form of visual processing.
However, reliably using AI means correctly dealing with unexpected situations or inputs and the accuracy of an AI system relies on using tremendous amounts of data. This is why many AI (and machine learning) systems are emerging as cloud-based services. It is more feasible to process the millions or billions of visual examples in the cloud necessary to sufficiently train the system. More training data means having the ability to consider more variables and reach more detailed conclusions. It's safe to say that cloud computing is what has made AI commercially viable today.
Q: What are the quantifiable benefits of integrating AI in manufacturing?
Robotics is the most obvious. The same technology being used to help self-driving vehicles navigate or keeping a Roomba vacuum from running over your cat is making its way to the shop floor. Robots aren't new to manufacturing, but they have traditionally been very expensive to deploy. Some models required magnets embedded in the floor to serve as tracks for guiding the machines, whose routes and tasks all had to be preprogrammed. This means any changes to the plant layout, an expected pallet of material in a corridor or new manufacturing processes required reprogramming the robotic staff.
But companies like Adept changed the game, with robots that sense the plant layout automatically. These robots can walk the plant to discover all of the areas. Once a map has been created, the human staff can provide the names of important areas, which then makes it easy to instruct a robot to fetch material from one place and deliver it to another. If a new obstacle is discovered - like an unexpected pallet or a new copier - the robots find another route and note the change for future tasks.
AI will help manufactures stay competitive, reduce costs, optimise capital employed and provide a better environment for their employees and service to their customers.
Another benefit is safer working environments. Using inexpensive cameras, AI can detect and track workers entering hazardous areas and even ensure protective gear is being worn. AI can also co-ordinate processes to ensure correct procedures are used.
Q: What aspects of the supply chain are being revolutionised by AI?
Large manufacturing enterprises are beginning to use AI to make material purchasing and allocation decisions. The classic manufacturing resource planning (MRP) process was invented at a time when the sales forecast, inventory levels, and existing purchase commitments were planned around longer date horizons. But the economy has evolved around shorter invention cycles, globalization, and sustainability, and mass personalisation (to name a few). So, both manufacturers and distributors have to become more agile, which means planning can be a daily process for many companies. AI can be used to predict micro-level lulls or spikes in demand, which in turn can determine the best routing for raw materials. This is exactly the rationale Merck is using to automate its factories.1 The same approach can work for any company, especially those with many product lines and complex manufacturing processes.
Factories of the future are taking MRP to the next level. Machine learning models suggest changes to planning parameters, lead times, and inventory stocking levels, and predict quality issues and down chain disruptions to both lead-time and price insulating the end customer and supporting their expectation of immediate gratification.
AI will also help set better expectations, for manufacturers on delivery dates and volumes based on capacity along with planned and unplanned downtime. And AI can help companies decide what to do with spare capacity, like producing seasonal items early that can be wholesaled to retail outlets at lower cost later in the year.
Q: Which specific industries have the highest AI adoption?
Logistics companies embraced AI once it was clear that freight routing was easy to optimise using the detailed mapping, traffic, shipping, and weather information, which has all materialised online in the last few years. But the biggest push recently for AI has been in healthcare. One much-cited example is the use of IBM's Watson, which can identify very early stage diseases by examining tomography and other data.
Typically, as supervised AI requires good data and good training, most early success will come in industries that use common data. For example, logistics companies all use the same types of road map, weather and traffic data. Likewise, virtually all retailers use the same Universal Product Code (UPC) to identify products, which mean AI techniques applied to UPC data benefits a large number of customers, and this will drive AI companies to build solutions for retailers. In other words, industries adopt AI when the solutions begin to emerge. So, industries with high-quality data that is readily available will have AI solutions ready sooner than others.
Q: How receptive are Epicor customers when it comes to embracing AI?
Many of our customers are mid-market enterprises that are very receptive to apply new technologies once it's clear what problems are solved and the solutions are affordable to deploy. For AI, there is an added challenge of building trust in the system to a point where people let go of their long-standing gut instincts, which possibly have served them well. As it becomes necessary to consider more variables on a continuous basis, using AI-enriched software will eventually become the best way to get accurate decisions made. Epicor customers choose us as a business partner because they wanted to grow and use the best technology platform to do so. At some point, companies will have no choice but to automate more of the decision-making process.
Q: How is the increased amount of data being produced from AI being used by various departments within a manufacturing company?
Ray Wang at Constellation Research summarises the maturity model of AI very well. It aligns well with how we are applying AI to our software solutions and is a seven-stage approach. AI is paving the way to allow us to make products people want in the way they want them, instead of making products and spending our time trying to make people want them.
AI can help with management decision support and planning, particularly in some service sectors where understanding population centres and demographics is key to maximising growth. It is also being used to help identify where the best placement globally to service customers is by capturing and reasoning over the many complex interdependencies manufactures deal with along with optimisation of facilities.
Q: How is AI supporting manufacturing workers and what specific roles are feeling the most benefit?
AI is getting quite good at understanding natural language and translating it, which means it will become easier for workers and managers to interact with software more naturally. Software users prefer to search for things rather than navigate a complex menu. In short, AI will help workers get things done in a more intuitive way, which leads to higher productivity and fewer errors.
Q: Is AI replacing jobs within the manufacturing industry? What specific job roles will we see replaced as AI adoption increases?
There is a lot of conjecture about this topic. Many modern manufacturing facilities run with very few employees already and this is why manufacturing productivity is actually far higher than most people realise. In the near term, autonomous vehicles may, for example, replace forklift drivers. Better computer vision software can make it easier for fewer staff to ensure quality on a mass scale. But these basic automation trends have been in place for some time. The next wave of automation will probably impact roles that analyse data and recommend actions to optimise the business, from design to operations and service. If AI systems can analyse images and correlate data from many sources, then it's possible for a computer to design the next popular clothing line or at least predict which lines will be successful.
People whose primary role is to interact with other people - sales, support, middle management - are today considered safe from being displaced by AI. But that's mainly because AI hasn't quite reached the point where it can converse with humans reliably in general cases.
Product design will also be heavily disrupted by AI as we start seeing generative design tools where the problem is defined and all of the problem space is explored computationally, amplifying our cognitive abilities. Augmented collaboration will be the working environment of the future. Human robot collaboration will be more gesture-based, where workers will be doing the things humans are good at - perception, awareness and decision-making - while robots will be doing the things they are currently good at, repetitive tasks with precision.
Q: What trends do you see emerging?
AI will impact manufacturing in ways we have not yet imagined. However, we can already look at some more obvious examples.
The continued improvement in computer vision (CV) has long been used for quality assurance by detecting product defects in real-time. But now that manufacturing involves more data than ever - coupled with the fact that plant managers do not want to pay staff to enter data - AI with computer vision can streamline how data gets captured. A factory worker should be able to take raw materials stock from the shelf and have the inventory transaction created automatically based on a camera observing the process. This will be the natural user interface, just completing the task at hand not inputting or scanning things into a system.
The second area that AI will impact is with the Internet of things (IOT) or cyber physical systems. IOT is remarkable in that the basic technology is being deployed rapidly, even though the outcomes and security aspects haven't really been thought through. Having detailed operational sensors in finished goods is clearly going to change markets and production tactics. IOT will provide a way to deliver supplies and services to customers who might not realise they are needed. In addition, IOT can send detailed telemetry back to producers and distributors to analyse quality and factors that might drive failures. In short, IOT is an incoming tsunami of data that AI can use to reason over and evolve. This will help augmented generative design processes where products are reimagined in ways more akin to evolution.
Q: Finally, what one piece of advice would you pass on to someone considering where AI fits in their business?
AI is not yet a turnkey solution. You don't buy it off the self and use it; you infuse it in everything you do to augment your business and products and unlock potential for future business growth. My advice is to start by looking at solutions that help your staff make faster and more accurate decisions.
1 Nash, Kim S, "Merck Deploys AI for 'Self-Driving' Supply Chain", The Wall Street Journal.com. Dec 20, 2016. http://blogs.wsj.com/cio/2016/12/20/merck-deploys-ai-for-self-driving-supply-chain/
Share