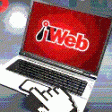
Understanding data science competencies is crucial for educating and training a competitive workforce for the rapidly growing global data market.
This is according to research undertaken by the University of Pretoria’s Rennie Naidoo (associate professor at the School of IT, Department of Informatics), Marie Hattingh, Linda Marshall and Marlene Holmner.
A data science competency model is beneficial for educators, government agencies, students, employers, employees and human resource development professionals. This is especially relevant because of the implication of the technologies associated with the fourth industrial revolution, such as data science analytical tools and machine learning.
A model grounded in theory and supported by empirical evidence is crucial in closing the skills gap, thereby improving the quality and competitiveness of the South African workforce in the rapidly growing global data market, the university established.
According to Naidoo, the research is one of the first of its kind to make use of the KSAO model to understand the competencies of a data scientist.
The acronym KSAO stands for knowledge, skills, abilities and other personal characteristics that an individual requires to perform a job. A data science competency is defined as a collection of knowledge, skills, abilities and other personal characteristics that are required by an individual or team when using insights that are systematically extracted from data to solve a problem in a given context.
To develop this model, the academics conducted a systematic literature review to identify the competencies that are essential to developing a globally competitive workforce in the field of data science.
The analysis revealed six major competencies: technical, organisational, analytical, ethical and regulatory, cognitive and social; and seven core disciplines: business management, computer science, information science, information systems, mathematics, statistics and natural science.
Figure one illustrates the representation of research per competency and discipline. The size of the bubble proportionally represents the number of papers addressing the competence per discipline.
Figure two shows that the most prominent relationship exists between the technical competencies in the computer science discipline, followed by organisational competencies within the business management discipline.
The least prominent relationships exist between ethics and regulatory competencies and the disciplines of information systems, statistics and natural science and the organisational competence and the disciplines of information science, mathematics and statistics.
Each category in figure two is proportioned to show the current importance of a specific competency. For example, almost double the amount of attention is being paid to cognitive competencies than for the social competencies. All competencies fall within the organisational domain, emphasising the role of the data science professional within an organisational context.
The analysis also identified associated sub-competencies to the six major data science competencies:
- Organisational: Contextual knowledge, domain knowledge, management skills and strategic thinking;
- Technical: Big data management, computational intelligence, computer architecture, computer networking, computer programming, computer security, data visualisation, statistics, software development, mathematical modelling;
- Analytical: Understanding the business context and supporting the technical competence;
- Ethical and regulatory: Information ethical issues, social responsibility, regulatory and policy issues;
- Cognitive: Critical thinking, problem solving, ‘solutioneering’, visual intelligence, self-management; and
- Social: Communication, collaboration and people aspects.
Recommendations:
Focus on building a team-based competency: Individual talent is scarce. Therefore, a team of data scientists that complement each other’s competencies is more likely to generate superior performance and competitive advantage in the organisation. This is crucial, given the shortage of data scientists in South Africa.
Recognise that data science competencies are multi-disciplinary: Data science competencies require varying levels of expertise. For example, a data scientist can have an advanced statistical competency with a novice programming competency level. It is not expected (and unlikely) that a data scientist can be an expert in all disciplines.
Do not underestimate the value of ethical, regulatory and social competencies: While it is understandable that efforts are being placed on the technical, analytical and cognitive competencies, attention also needs to be paid to ethical, regulatory and social competencies.
These recommendations provide a crucial starting point to improving the performance of your data science team, say the researchers.
* This study was conducted by the Data Science Competency Research Group at the University of Pretoria’s School of Information Technology. The team members who contributed jointly to this research effort are Dr Marie Hattingh, Prof Rennie Naidoo, Dr Marlene Holmner and Dr Linda Marshall.
Source: Hattingh, M., Marshall, L., Holmner, M. and Naidoo, R., Data Science Competency in Organisations: A Systematic Review and Unified Model, Upcoming paper in SAICSIT 2019.
Share