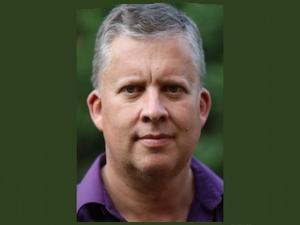
Moving data can be complex, challenging and add high levels of risk to an overall migration or implementation project. However, a successful data migration can enable companies to gain valuable business benefits such as increased productivity, reduced costs and greater customer satisfaction.
Yet, for those of you who have ever undertaken a data migration project, you'll already know how critical the effective reconciliation of data is to overall project success. It's widely recognised that a high proportion of IT projects fail, but a white paper on data migration published by Bloor Research found that there was a 38% failure rate of data migration projects.
Plotting the 'happy path'
There are many things that can contribute to data migration project failure including late planning, but for this article we are focusing on data reconciliation. All too often in projects the 'happy path' is plotted and generally involves perfection in every element, including the use of small amounts of high quality and complete data.
In a typical data migration project, the ETL (extract, transform and load) process is likely to include a number of interactions such as high-level data counts as well as manual spot tests on data, but the true state and quality of the data is frequently not really known until it is loaded onto the new system.
Barry MacDougall, Service Delivery Director at JMR Software, warns of the risks of poor quality data and says: "Where the extent of dirty data is unknown it could present a high risk, particularly where it prevents a load-in to a target system and especially if the extent of the problem results in unexpectedly high data-related issues. Resolving any kind of data issues is likely to impact the delivery timelines and cause a delay in completing the project."
High visibility and reconciliation of data in flight is critical
As data continues to grow in value, volume and the velocity, ensuring your data is as correct and complete as possible when moving to the new world is critical to your data migration success.
"We recognised years ago that every single piece of data to be migrated is important and therefore needs our attention. Any good data migration solution should have a built-in and detailed reconciliation capability that also enables high levels of automation," emphasises MacDougall.
How do you know the extent of your data quality problem?
"In order to fix it, you need to understand the size and extent of the data quality problem before you move the data", suggests MacDougall, "we recommend reconciling data down to an individual field level and at every stage in the process, which means that you can check you have the volume, but you are also able to check the quality of data at field level and its completeness in flight."
In other words, reconciling at a field level will help you to identify poor data quality that can then be fixed in one of two ways; feeding back to the business to manually clean the data at source, or automate elements of cleansing through the transformation process.
A good data migration solution should enable some cleansing to be incorporated into a data migration project by agreeing cleansing rules that can then be automated during transformation. These Rules should be defined during the mapping phase of the data migration project and with the JMR Software's approach can be used in multiple testing cycles to improve the dataset.
MacDougall says: "For over 30 years we have been working with large enterprises and legacy systems, particularly in financial services, who recognise the critical nature and importance of their data as they migrate. The only way to remove the majority of risk from a data migration project is by carrying out detailed reconciliation at every stage."
In conclusion, MacDougall says: "Inaccurate, incomplete or poor-quality data discovered at User Acceptance Testing will lead to dissatisfied users and is likely to have an impact on the perception of the overall application or new system being deployed. It will also ultimately lead to a large amount of data cleansing work as well as a potential production delay. Full and automated reconciliation of data is critical as data migration demands continue to grow."
Share