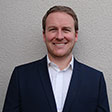
As businesses become more data-driven, with more data available than ever before, a focus on data management naturally becomes more important.
The ability to manage business data intelligently starts by focusing on making the data useful. In other words, data services should be designed around the data (what it is, how it arrives and where it is stored) to enable insights to be derived from the data, and to support business processes.
The ongoing open source revolution and cloud boom has enabled a plethora of technical capabilities, quicker than ever. However, a challenging economy and the reality of a skills shortage mean effort must still be focused on key business drivers.
To intelligently manage data, a business should have an effective data strategy in place, which aligns with the business strategy.
Management aspects such as data governance, data architecture, master data management, data quality and so on, follow naturally once the data strategy is known. From the data strategy come the principles and standards which then guide data management decisions.
By way of example, consider one data strategy goal: to provide 'a single view of the customer'.
Starting with the basics, a business needs to get the right data to the right place. The 'right place' is one (or usually more) subject matter experts or systems which can action the data insights. This speaks to data visibility and communication as a function of data management. The business must understand what it is looking at in order to make decisions.
Data management cannot be solely about getting 'all the things in one place' but rather about how to get value from all the things.
An enterprise data model should be built to provide communication around the data meaning. Data marts could provide analytics stores, which can suffice for the 'single version of the truth'. Note that the business doesn't necessarily need one system to house the data, or just one copy of the data; rather, it might want batch and real-time components, or a variety of data formats, which means it may need several capabilities. A data model should be established and implemented, so that the 'single view' can be produced from its constituents.
Once the business has a data model which provides communication around the data, a good data management strategy would consider the protection of the data. Non-functional aspects such as security (access control in particular), backups and replication (availability), and reliability of data services to consumers, need to be considered here.
Since these are based on a consistent data strategy, the business would be able to implement patterns which comply to the strategy and can be re-used. Re-use encourages automation for deployment and management of these patterns in future, further accelerating value extraction from the data pipelines.
Businesses must avoid falling for the latest hype cycle based on isolated industry success stories. Data lakes, artificial intelligence, cloud, real-time streaming, machine learning, etc, are tools which enable effective, or ineffective, data management. The difference being only the value gained from the data insights upon application of the tools.
Take the data lake concept as an example, being a central data store for consumers to access any and all raw data. This alone cannot enable insights and is at risk of becoming a petabyte-sized data swamp.
Data management cannot be solely about getting 'all the things in one place' but rather about how to get value from all the things. A business needs to consider data arrival, storage and usability of the data lake, to realise this value. Once the data services estate matures, the business may want to consider data virtualisation; especially SQL-based as SQL becomes more ubiquitous.
Intelligent data management is especially crucial if the business plans to give users unlimited scalability at their fingertips; eg, with cloud services. Scalability cannot always compensate for poor design, at least on a basis of cost-effectiveness.
A data dictionary, subject marts and effective tooling can help to intelligently manage data and provide structure and ease of use. By streamlining the process to access, utilise and trust data, effective data management then becomes a self-fulfilling paradigm as the barriers to use and maintain the data are broken down.
Data quality and metadata tooling are ultimately only as effective as the subject matter experts and systems that can use them for action. Intelligent data management is about making it easy to use these tools. Consider starting with quality and metadata basics, and work from there rather than starting with larger frameworks with a less transparent path to value.
Finally, adding to the data estate should be a collaborative process where users are encouraged and enabled to re-use data and contribute back. This speaks not only to data management but also to having tools that enable collaboration; however, this is a topic on its own.
Suffice to say, intelligent data management has several focus areas. Ultimately, it is about collating, storing, maintaining, availing, automating and presenting data, in an intelligent manner, to enable business decision-making.
Share